![[BKEYWORD-0-3] The Drug Target And Mechanism Of Action](https://philschatz.com/microbiology-book/resources/OSC_Microbio_14_03_ART.jpg)
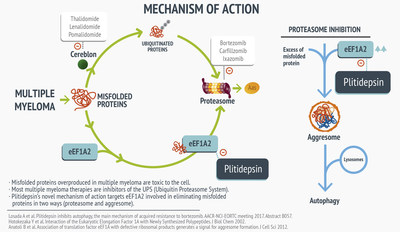
Thank you for visiting nature. You are using a browser version with limited support for CSS. To obtain the best experience, we recommend you use a more up to date browser or turn off compatibility mode in Internet Explorer. In the meantime, to ensure continued support, we are displaying the site without styles and JavaScript. Phenotype-based compound screening has advantages over target-based drug discovery, but is unscalable and lacks understanding of mechanism of drug action.
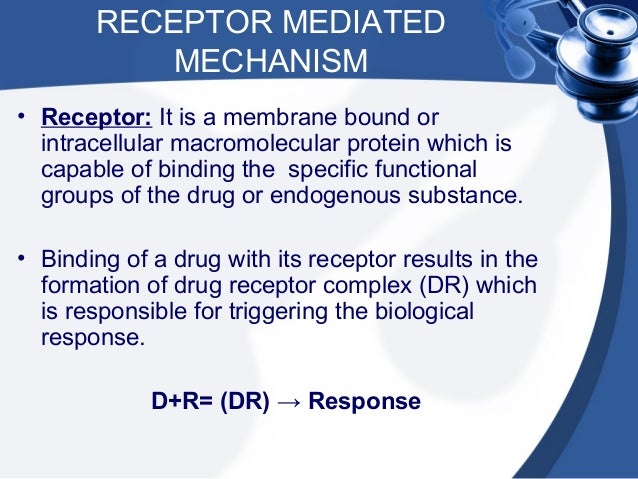
A chemical-induced gene expression profile provides a mechanistic signature of phenotypic response; however, the use of such data is limited by their sparseness, unreliability and relatively low throughput. Few methods can perform phenotype-based de novo chemical compound screening.
Post navigation
Here we propose a mechanism-driven neural network-based method, DeepCE—which utilizes a graph neural network and multihead attention mechanism to model chemical substructure—gene and gene—gene associations—for predicting the differential gene expression profile perturbed by de novo chemicals. Moreover, we propose a novel data augmentation method that extracts useful information from unreliable experiments in the L dataset. The experimental results show that DeepCE achieves superior performances to state-of-the-art methods.
The effectiveness of gene expression profiles generated from DeepCE is further supported by comparing them with observed data for downstream classification tasks. DeepCE thus provides a potentially powerful framework for robust predictive modelling by utilizing noisy omics data and screening novel chemicals for the modulation of a systemic response to disease. Target-based high-throughput screening dominates the Mecnanism drug discovery process.
It has been the focus of computer-aided drug discovery for decades, including recent applications of deep read article however, the readout from the modulation of a single protein by a chemical is poorly correlated with organism-level therapeutic effects or side effects. As a result, the failure rate from a lead compound generated from the target-based screening to approved drug is high.
Phenotype-based screening has created renewed interests for identifying cell-active compounds but suffered from low throughput and difficulty in target deconvolution. A high-throughput, mechanism-driven Mechanixm compound screening method will therefore facilitate drug discovery and development. Gene expression profiling has been widely used to characterize cellular and organismal phenotypes. Systematic analysis of genome-wide gene expression of chemical perturbations on human cell lines has led to considerable improvements in drug discovery and systems pharmacology.
Navigation menu
In particular, gene expression profiling can be applied to drug repurposing 1234discovering drug mechanisms 5lead compound identification 6 and predicting side effects for preclinical compounds 7. The L dataset and its normalization versions 10 were recently widely used in drug repurposing and discovery 11 Despite these successes, there are several major problems when utilizing L First, although the number of gene expression profiles is much larger than that in CMap, many missing expression values remain in the vast combinatorial space of chemicals and cell lines. Second, there are hundreds of millions of drug-like, purchasable chemicals that are potential drug candidates ]
Excuse, I have removed this idea :)