Linear Programming in Healthcare Systems Video
Linear Programming Linear Programming in Healthcare Systems.In this paper, we present new preconditioners based on the incomplete Cholesky factorization and on the splitting preconditioner. In the first approach, we consider the interior point methods that are very efficient for solving linear programming problems. The results of the numerical tests for this problem present satisfactory results in relation to the time and number of iterations. In the second approach, we apply a new preconditioner in compressive sensing CS problems, which is an efficient technique to acquire and reconstruct signal. An approach for solving this problem is the primal-dual Newton conjugate gradients.
We present a new preconditioner, in the construction of which we exploited the fact that close to a solution we can split the variables into two groups and the matrices satisfy certain properties, as Linear Programming in Healthcare Systems in a method known from the literature Fountoulakis Once the preconditioner exploiting these features has been computed, we apply an incomplete Cholesky factorization on it, and use the factor found as the true preconditioner.
Therefore, the new preconditioner is the result of the combination of two preconditioners. The results obtained are satisfactory in relation to the time and the quality of the reconstructed image.
Linear Programming in Healthcare Systems
Linear programming problems can be found in a variety of contexts, such as fleet and Linear Programming in Healthcare Systems logistics planning, operational strategies in mining, steel, agriculture, and others. Among the approaches in the literature for solving linear programming problems, in the first section of the paper, we use the predictor-corrector interior point method. A linear optimization problem consists of minimizing or maximizing a linear objective function, subject to a finite set of linear constraints. Restrictions can be either equality or inequality. The standard form of a linear optimization problem is called a primal problem and is given by:. We know that the matrix of the linear system can be ill-conditioned, so the use of the preconditioners is mandatory when using iterative methods. We present a new preconditioner for linear programming https://amazonia.fiocruz.br/scdp/blog/culture-and-selfaeesteem/alcohol-advertising-essay.php, which we will call the type splitting factor and present numerical results.
An efficient technique in acquiring and reconstructing signals is compressive sensing CSalso known as compressive sampling [ 5 ]. Compressive sensing is applied in the areas of photography [ 13 ], magnetic resonance [ 16 ], and tomography [ 9 ], among others. Linear Programming in Healthcare Systems new method applied to compressive sensing Relationship Between Intelligence And Success presented in this paper. We present our preconditioner applied to the compressive sensing problem.
Following the same reasoning presented for linear programming problems, we will apply the incomplete Cholesky factorization in the preconditioner developed in [ 10 ], considering that like the splitting preconditioner, it achieves better performance close to a solution. Numerical experiments related to this new preconditioner are also presented. The results obtained, both for linear programming problems and compression sensing problems, are satisfactory concerning the preconditioners already known in the literature. In the interior point methods, we obtain a linear system corresponding to the Newton method applied to the optimality conditions of the problem.
Non Linear And Dynamic System Analysis
The solution of the system can be done by means of direct methods, such as LU and Cholesky factorizations or iterative methods, such as the conjugate gradient method CGM. The Cholesky factorization [ 11 ] is the most often used method for the linear system solution. The computation of the factors can be very expensive, since in problems with sparse matrices its structure can Sysfems affected with fill-in, that is, an appearance of extra nonzero entries in the Cholesky factor. Equipped with appropriate preconditioners, iterative methods become a good alternative.
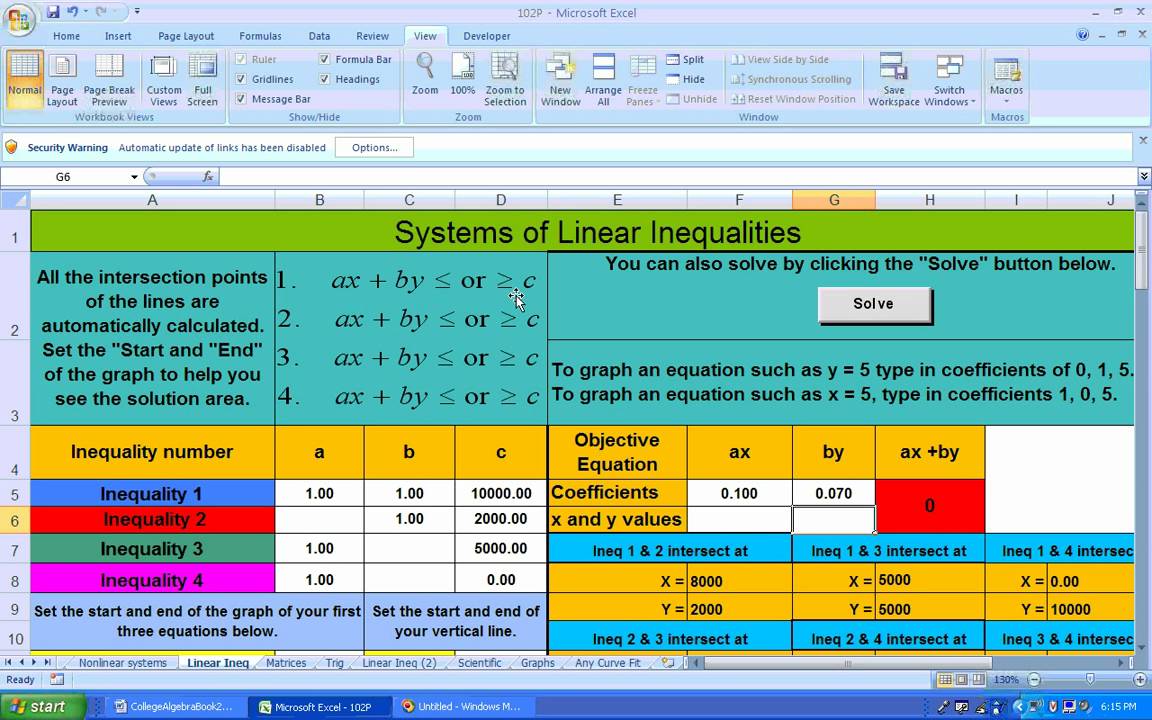
As the matrix of Lihear system is positive definite, we apply the conjugate gradient method which has a good performance when the matrices are well conditioned; in case this does not occur, preconditioning becomes necessary. The splitting preconditioner M M T [ 18 ] arose from interior point methods that use iterative approaches, in particular, the conjugate gradient method. The construction of this preconditioner M M T is based on the behavior of matrix D in the final iterations of the interior point method. Consider D kthe matrix D corresponding to k -th iteration, the elements of D k are given by:.]
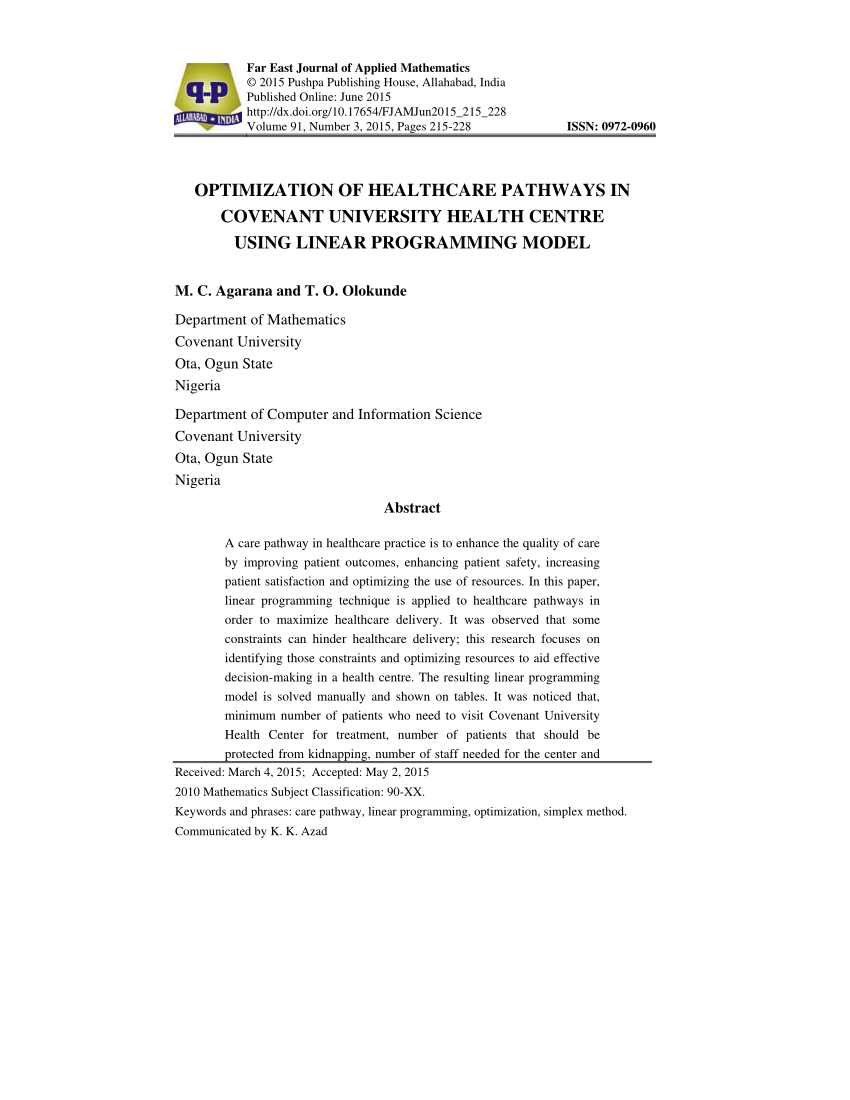
One thought on “Linear Programming in Healthcare Systems”