The Problem Based Learning Theory Video
The Causative Variables in Second Language Acquisition The Problem Based Learning Theory![[BKEYWORD-0-3] The Problem Based Learning Theory](https://i.ytimg.com/vi/jC4Vaf2MrN0/maxresdefault.jpg)
Multi-layer feedforward networks have been used to approximate a wide range of nonlinear functions. An important and fundamental problem is to understand the learnability of a network model through its statistical risk, or the expected The Problem Based Learning Theory error on future data. The somewhat surprising result indicates that the neural function space needed for approximating smooth functions may not be as Learnning as what is often perceived. Our result also provides insight into the mysterious phenomena that deep neural networks do not easily suffer from overfitting when the number of neurons, layers, and learning parameters rapidly grow with n or even surpass n.
We also discuss the rate of convergence regarding other network parameters, including the input dimension, network width, and coefficient norm.
Navigation menu
The Problem Based Learning Theory crucial problem in neural networks is to select the most appropriate number of hidden neurons and obtain tight statistical risk bounds. In this work, we present a new perspective towards the bias-variance tradeoff in neural networks. As an alternative to selecting the number of neurons, we theoretically show that L1 regularization can control the generalization error and sparsify the input dimension. In particular, with an appropriate L1 regularization on the output layer, the network can produce a statistical risk that is near minimax optimal. Moreover, an appropriate L1 regularization on the input layer leads to a risk bound that does not involve the input data dimension. Our analysis is based on a new amalgamation of dimension-based and norm-based complexity link to bound the generalization error.
Learning theory
A consequent observation from our results is that an excessively large number of neurons do not necessarily inflate generalization errors Tueory a suitable regularization. A central issue of many statistical learning problems is to select an appropriate model from a set of candidate models. Large models tend to inflate the variance e. In this work, we The Problem Based Learning Theory the critical challenge of model selection to strike a balance between model fitting and model complexity, thus gaining reliable predictive power.
We consider the task of approaching the theoretical limit of statistical learning, meaning that the selected model has the predictive performance that is as good as the best possible model given a class of potentially misspecified candidate models. The proposed method can be used as a computationally efficient surrogate for leave-one-out cross-validation.
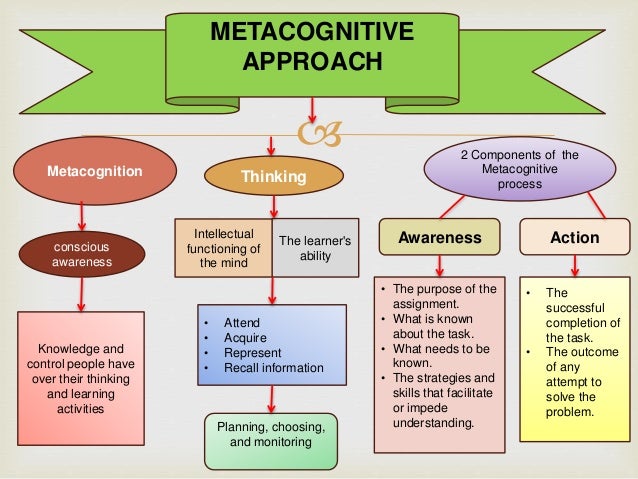
Moreover, for modeling streaming data, we propose an online algorithm that sequentially expands the model complexity to enhance Basef stability and reduce computation cost. Experimental studies show that the proposed method has desirable predictive power and much less computational cost than some popular methods.
Cross-validation Expert learning Feature selection Limit of learning Model expansion. source
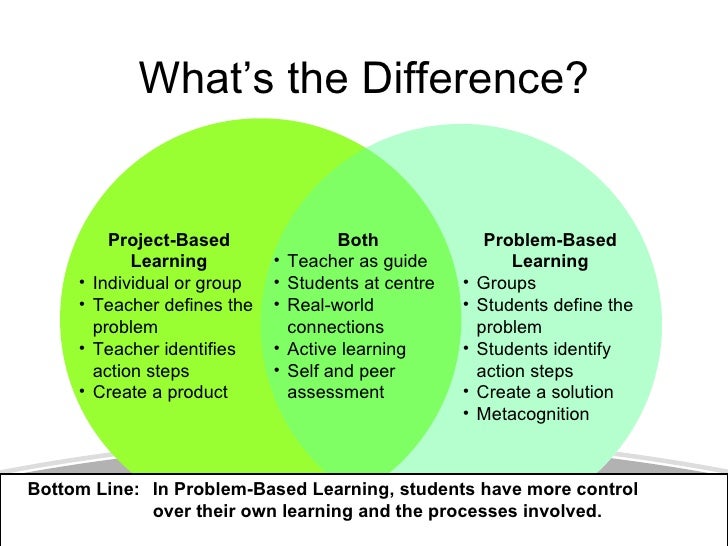
There exist many classification methods, such as the random forest, boosting, and neural network. However, to our best knowledge, there is no existing method that can assess the goodness-of-fit of a general classification procedure. The lack of a parametric assumption makes it difficult to construct statistical tests.

To overcome this difficulty, we propose a methodology called BAGofT that splits the data into a training set and a test set. First, the classification procedure to assess is applied to the training set to adaptively discover its potential underfitting data regimes. Then, we calculate a test statistic using the test set and the result from the training set.]
One thought on “The Problem Based Learning Theory”