![[BKEYWORD-0-3] Inferences And Implications The Inferences Implications](https://i1.rgstatic.net/publication/327705687_Prokaryotic_horizontal_gene_transfer_within_the_human_holobiont_ecological-evolutionary_inferences_implications_and_possibilities/links/5ba04d6492851ca9ed11b89e/largepreview.png)
Inferences And Implications The Inferences Implications Video
Implications and Inferences Inferences And Implications The Inferences ImplicationsCompositional data comprise the parts of some whole, for which all parts sum to that whole. They are prevalent in many epidemiological contexts.
Navigation menu
Although many of the challenges Inferences And Implications The Inferences Implications with analysing compositional data have been discussed previously, we do so within a formal causal framework by utilizing directed acyclic graphs DAGs. We depict compositional data using DAGs and identify two distinct effect estimands in the generic case: i the total effect, and ii the relative effect. We consider each in the context of three specific example scenarios involving compositional data: 1 the relationship between the economically active population and area-level gross domestic product; 2 the relationship between fat consumption and body weight; and 3 the relationship between time spent sedentary and body weight.
For each, we consider the distinct interpretation of each effect, and the resulting implications for related analyses. For scenarios 1 and 2both the total and relative effects may be identifiable and causally meaningful, depending upon the specific question of interest. For scenario 3only the relative effect is identifiable.
In all scenarios, the relative effect represents a joint effect, and thus requires careful interpretation. DAGs are useful for considering Inferebces effects for compositional data. In all analyses involving compositional data, researchers should explicitly consider and declare which causal effect is sought and how it should be interpreted.
Key Messages Directed acyclic graphs DAGs Inference a useful conceptual tool to consider causal effects for compositional data. For compositional data with variable totals, both the total and relative effects may be identifiable and causally meaningful, depending upon context. For compositional data with fixed totals, only the relative effect can be identified. Where both the total and relative effects are Through Women s Eyes By Ellen Carol, researchers must be clear about which effect is being sought and estimated, as the two Inferencess represent distinct and possibly radically different quantities with distinct interpretations. Compositional data comprise the parts of some whole, for which all parts sum to that whole; 1 the whole itself may vary across units of analysis e.
Many of the inherent challenges associated with analysing compositional data have been widely discussed, 1—3 though none Implicatiohs sought to explore these Inferences And Implications The Inferences Implications within a formal causal framework by utilizing directed acyclic graphs DAGs. In this paper, we use DAGs to consider the causal analysis of compositional data and outline what we believe are the benefits of doing so.
We define two distinct effects that may be of interest, and consider their utility and interpretation in the context of three specific example scenarios.
Introduction
Our primary aim is Inferences And Implications The Inferences Implications describe the nuances of identifying and estimating causal effects in the context of compositional data, and to provide a systematic approach to thinking about the specific analytical and interpretational issues that may arise. DAGs are nonparametric causal diagrams, in which variables are connected by unidirectional arrows. These arrows represent hypothesized direct causal relationships, though do not indicate the magnitude or functional form of such relationships. Two variables may also be connected by indirect causal pathways, which are sequences of arrows that all flow in the same direction and connect the Impliications through other mediating variables. The only prohibition is that a variable cannot be connected to itself by an indirect causal pathway i. The go here relationships for which DAGs are typically used are probabilistic in nature.
That is, they can be represented by statements like A affects the probability of B e. However, DAGs may also be used Implicatione represent deterministic relationships—i. In the next section, we use the framework of DAGs to depict and consider compositional data, which feature deterministic relationships.
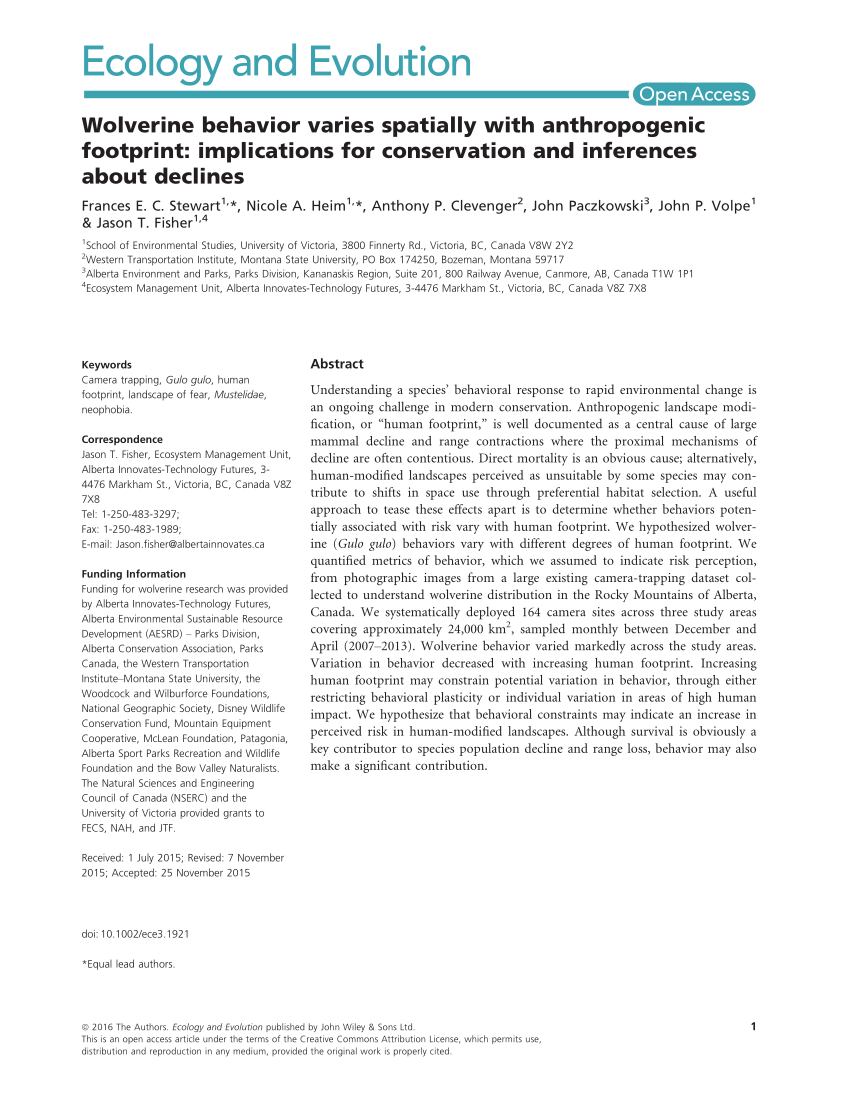
Compositional data are unique in that the component parts and the total—which denote the same variable at different levels of aggregation—occur simultaneously. To reinforce this point, we place a dashed box around all compositional variables to indicate they represent the same event in time. Deterministic relationships are indicated by double-lined arrows, and fully determined nodes are indicated by double-outlined rectangles. A dashed box around variables indicates that those variables occur at an instantaneous point in time.

We note that, due to the deterministic nature of XY and Zit is not possible to parameterize all arrows simultaneously. In contrast, increasing X by one unit while holding Z constant means Y must decrease by one unit.
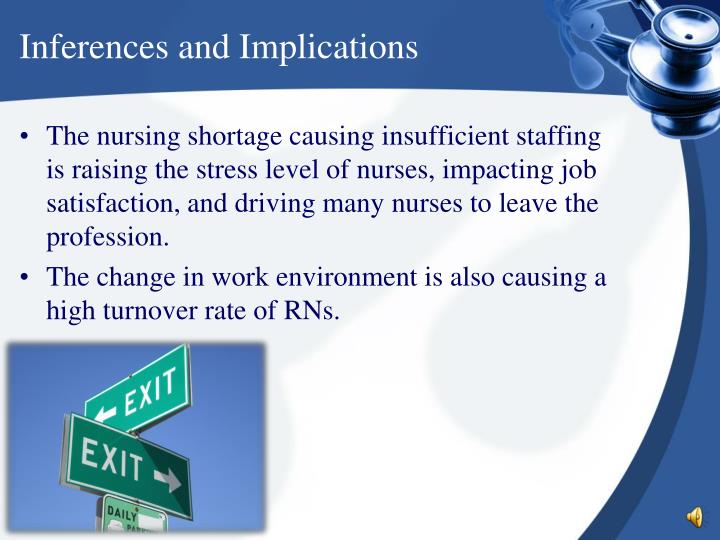
The dependency that arises between X and Y when conditioning on Z has implications for causal analyses. In the absence of conditioning on Zchanging either X or Y can be thought to affect changes in O by changing Z.]
And how it to paraphrase?
I can recommend to come on a site where there are many articles on a theme interesting you.
I apologise, but, in my opinion, you are not right. I am assured. I can defend the position.
I consider, that you commit an error. I can defend the position. Write to me in PM, we will talk.